December 2, 2022
Some of the most promising green energy technologies are hindered by their durability. As these technologies are quite new, there is a dearth of research into device failure mechanisms and effective service lifetimes. These unknowns constitute risks to investors and barriers to commercialization.
ChemE’s are applying their AI skill set to identify, understand and predict the failure modes of perovskite solar cells and solid oxide fuel cells. New insights may enable those devices to be deployed commercially with predictable warranty models and minimal down-time and revenue loss.
Perovskite solar cells are one of the most rapidly advancing solar technologies: they have power conversion efficiencies on par with silicon solar cells while also being lighter weight and more flexible. They also have shorter energy payback times and lower manufacturing costs. ChemE professors Hugh Hillhouse and David Beck and UW statistics professor Marina Meila are working on a DOE-funded project to understand early indicators and mechanisms of perovskite degradation.
The researchers conducted high-throughput measurements of optoelectronic characteristics of perovskite films and devices under various environmental conditions. Analysis of the resulting dataset revealed critical physical processes limiting performance, including one that was previously unknown and uncharacterized.
From there, they developed machine learning models to make accurate predictions of cells’ service lifetimes based on composition and environmental conditions. They made their models, along with data acquisition and analysis methods, available as open science for others to use and further develop. The team believes their results can facilitate device design by reducing the need for expensive and time-consuming lifetime tests.
Related story
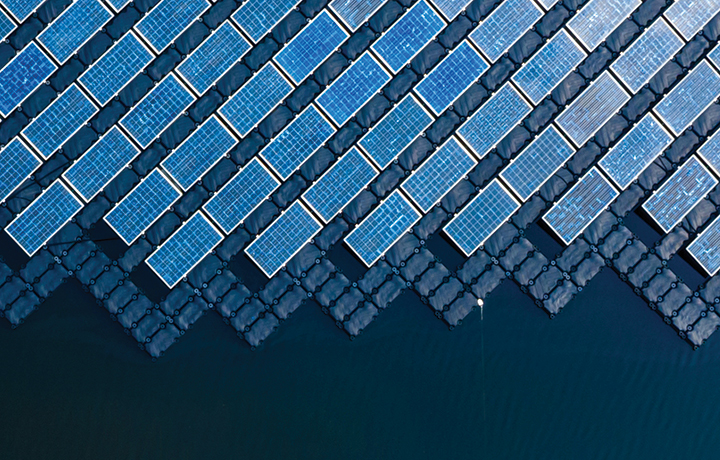
Champions of Sustainability
Discover how chemical engineers are leading the charge to a greener future
Elsewhere in ChemE, professor Stu Adler’s group is developing machine learning models to identify and preemptively predict the failure of solid-oxide fuel cells (SOFCs). SOFCs are more efficient, cheaper and easier to maintain than conventional batteries.
Fuel cell batteries are scalable by bundling individual cells into stacks. However, there’s a lack of diagnostic tools to detect and predict cell-level failures ahead of entire stack or system failures. To approach the problem, Adler’s group simulated the electrochemical impedance spectroscopy response of healthy and failing SOFC stacks and trained a machine learning model on the data. The model was able to detect and differentiate failure modes, as well as distinguish them from degradation due to long-term use. The results are an encouraging step toward making combustion-free power viable on a large scale.