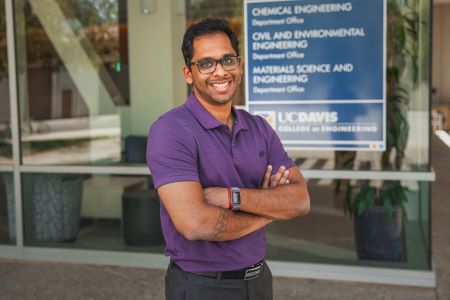
Ambarish Kulkarni
Assistant Professor
University of California, Davis
Data-Driven Molecular Modeling and the Design Novel Functional Materials
October 18
4:00:00 PM
PAA A110
Abstract
The democratization of machine learning (ML) and the advent of open-access data repositories have revolutionized materials science in the preceding decade. These advances have enabled high-throughput in-silico screening of large materials libraries, but the measurable impact on laboratory-scale development of functional materials and novel processes is still limited. Why? In part because of the disconnect between theoretical “models” and real-world experimental “samples.” For example, the promising active site environment of a catalyst (often predicted by theory) is often far different from what is synthesized in practice. A high-priority need is thus to develop integrated materials development workflows that bridge (1) computational materials design, (2) laboratory-scale materials synthesis, (3) unbiased experimental characterization, and (4) rapid performance evaluation. Recognizing these bottlenecks, this talk will present an overview of an agile, interdisciplinary, materials-agnostic research program led by Prof. Kulkarni at UC Davis. Topics include (1) cation-exchanged zeolites and the importance of local Al distributions, (2) electroactive metal-organic frameworks for fuel cells designed to circumvent scaling relationships, and (3) atomically dispersed transition metal catalytic sites stabilized on and within oxide surfaces (e.g., Pt/MgO). A central goal is to demonstrate unique insights provided by theory in collaboration with experimentalists. An example discussed in detail is the development of a theory-based approach to fitting Extended X-Ray Absorption Fine Structure (EXAFS) data, providing unprecedented structural detail and confidence in the local binding environment for “single-atom” catalysts like Pt/MgO. Another motivating case study is the use of surface-enhanced Raman spectroscopy (SERS) as a probe — illustrated with an ML-based classifier that minimizes user intervention and accelerates data collection and downstream analysis for early-stage disease diagnostics. Taken together, this talk will present a cohesive vision for the expanding role of theory and data science (used judiciously in conjunction with experimentation) to address challenges central to current and emerging technological needs.
Bio
Ambar Kulkarni is an Assistant Professor in the Department of Chemical Engineering at UC Davis. After receiving his B.S. from the Institute of Chemical Technology (formerly UDCT), Mumbai (India), he moved to the U.S. to complete his Ph.D. (Georgia Tech, with David Sholl) and postdoc (Stanford, with Jens Nørskov). Over the last three years, his research group has focused on combining advances in multiscale molecular modeling and machine learning with multifaceted experimental collaborations to addresses big challenges in catalysis, separations, and sensing. His research group has experience with a variety of functional materials (e.g., zeolites, metal-organic frameworks (MOFs), metals, oxides), simulation methodologies, and automated analyses of experimental results (e.g., X-ray absorption spectroscopy, NMR spectroscopy, surface-enhanced Raman spectroscopy). The Kulkarni group is supported through grants from the National Science Foundation (NSF), the Department of Energy (DOE), the Center for Data Science and Artificial Intelligence Research (CEDAR UC Davis), and industry partnerships. Kulkarni’s interdisciplinary research was recognized with the 2021 UC Davis College of Engineering Outstanding Junior Faculty Award for Excellence in Research. He received a 2021 NSF CAREER award for his work in designing MOFs for electrochemical applications. As the Inaugural UC Davis Graduate Mentor Fellow (2020-2022), Kulkarni has developed a series of mentorship workshops for UC Davis faculty aimed at improving the graduate student mentorship experience. When he is not staring at molecules on the computer screen, Ambar and his wife enjoy traveling to different countries, snowboarding, and playing with their dog.